Salesforce Data Cleansing: Quality, Accuracy Improvement
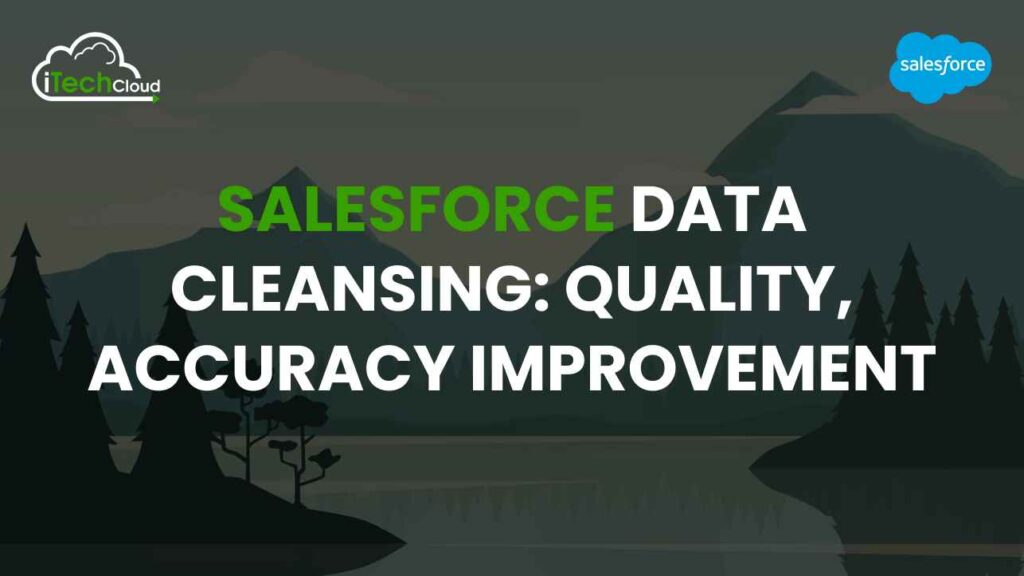
In the dynamic world of customer relationship management (CRM), maintaining high data quality is crucial. Salesforce Data Cleansing, as a leading CRM platform, enables businesses to manage customer interactions, track sales, and drive growth. However, the effectiveness of these processes hinges on the quality and accuracy of the data within Salesforce. Salesforce Data Cleansing is the process of identifying and correcting (or removing) corrupt or inaccurate records from a database. This blog explores the importance of data cleansing in Salesforce, the challenges associated with it, and the best practices to improve data quality and accuracy.
What is Salesforce Data Cleansing?
Salesforce Data Cleansing involves the process of identifying and rectifying inaccuracies, inconsistencies, and duplicates within the Salesforce database. It ensures data integrity, enhances data quality, and maximizes the effectiveness of sales, marketing, and customer service efforts. Techniques include deduplication, standardization, validation, and enrichment of data. Salesforce Data Cleansing, organizations can make informed decisions, improve productivity, and foster better customer relationships. It’s an essential step in maintaining a reliable and efficient CRM system.
Table of Contents
Challenges in Maintaining Salesforce Data Quality
Maintaining high-quality data within Salesforce is crucial for organizations to derive accurate insights, drive effective decision-making, and deliver superior customer experiences. However, numerous challenges can impede the ongoing effort to uphold data quality standards. Here’s an in-depth exploration of these challenges:
1. Data Entry Errors:
Human errors during data entry are common and can lead to inaccuracies in Salesforce records. Mistyped information, missing fields, and inconsistencies in formatting contribute to data quality issues. Without proper validation checks or data governance protocols, these errors propagate throughout the system, compromising its integrity.
2. Duplicate Records:
Duplicate records are a pervasive problem in Salesforce databases, resulting from various sources such as manual entry errors, system integrations, or data imports. Duplicate accounts, contacts, or leads lead to confusion, inefficiencies, and skewed analytics. Identifying and merging duplicates is a labor-intensive task that requires proactive measures and regular maintenance.
3. Data Decay:
Data in Salesforce can become outdated or irrelevant. Contact information changes, companies rebrand, and job titles evolve. Without periodic updates, stale data diminishes the effectiveness of marketing campaigns, sales outreach, and customer service interactions. Implementing strategies for data enrichment and regular data hygiene practices can mitigate data decay.
4. Integration Challenges:
Integrating Salesforce with other systems introduces complexities that can affect data quality. Inconsistent data formats, incompatible data models, and synchronization errors are common issues. Ensuring seamless data flow between Salesforce and other applications requires careful planning, robust integration tools, and ongoing monitoring to prevent data discrepancies.
5. Data Governance Lapses:
Inadequate data governance frameworks contribute to data quality issues in Salesforce. Without clear policies, roles, and responsibilities regarding data management, users may lack guidance on proper data handling practices. Data governance gaps can result in unauthorized access, data silos, and compliance risks, undermining the overall integrity of the Salesforce environment.
6. Lack of Standardization:
Inconsistent data formats and terminology hinder data quality efforts in Salesforce. Variations in naming conventions, abbreviations, and categorizations make it challenging to aggregate and analyze data accurately. Establishing standardized data fields, picklists, and validation rules promotes consistency and facilitates data quality improvements.
7. Data Security Concerns:
Data security breaches pose significant risks to Salesforce data quality. Unauthorized access, data theft, or malicious attacks can compromise the confidentiality, integrity, and availability of sensitive information. Implementing robust security measures, access controls, and encryption mechanisms is essential to safeguarding Salesforce data and maintaining trust with stakeholders.
8. Limited User Adoption:
Poor user adoption of Salesforce features and data entry protocols exacerbates data quality issues. Users may resist using Salesforce due to usability issues, lack of training, or perceived inefficiencies. Incomplete or inaccurate data entry by users impairs data quality and undermines the reliability of Salesforce as a CRM platform. Investing in user training, providing intuitive interfaces, and incentivizing data accuracy can improve user adoption and data quality.
9. Complex Data Models:
Complex data models in Salesforce orgs can complicate data quality management efforts. Custom objects, fields, and relationships increase the complexity of data governance, validation, and cleansing processes. Mapping data flows and dependencies becomes challenging, leading to potential inconsistencies and errors. Simplifying data models where possible and maintaining clear documentation can alleviate these challenges.
10. Scalability Issues:
As organizations scale their Salesforce implementations, managing data quality becomes more challenging. Increased data volume, user activity, and system complexity strain data management processes and resources. Scalability issues may manifest as performance degradation, delayed data updates, or resource constraints. Implementing scalable data quality solutions and infrastructure optimizations is essential to support growth without sacrificing data integrity.
Strategies for Salesforce Data Cleansing
Salesforce Data Cleansing in Salesforce is crucial to ensure the reliability, accuracy, and efficiency of your CRM system. Here are comprehensive strategies for Salesforce Data Cleansing in Salesforce, focusing on identifying, correcting, and preventing data quality issues.
1. Data Governance Policies
- Define Standards: Create standardized naming conventions, field formats, and data entry protocols to ensure consistency across the Salesforce database.
- Roles and Responsibilities: Assign specific roles for data stewards who oversee data quality and enforce governance policies.
- Compliance: Ensure adherence to regulatory requirements and industry standards to maintain data integrity and security.
2. Data Assessment and Profiling
- Data Audits: Regularly conduct data audits to identify and assess data quality issues such as duplicates, inaccuracies, and inconsistencies.
- Profiling Tools: Use data profiling tools to analyze data attributes, distribution, and patterns to detect anomalies and areas needing improvement.
3. Automated Data Cleaning Tools
- Deduplication Tools: Implement deduplication tools that automatically identify and merge duplicate records within Salesforce.
- Validation Rules: Create validation rules to enforce data entry standards and prevent erroneous data from being saved.
- Workflow Automation: Use Salesforce’s workflow automation capabilities to trigger data quality checks and corrective actions.
4. Manual Salesforce Data Cleansing Procedures
- Data Entry Guidelines: Train users on best practices for data entry to minimize errors and ensure high-quality data input.
- Periodic Reviews: Schedule regular manual reviews and updates of key data fields to ensure accuracy and completeness.
- Merge and Purge: Establish processes for merging duplicate records and purging outdated or irrelevant data.
5. Data Enrichment
- Third-Party Integration: Integrate with third-party data providers to enrich Salesforce records with additional information such as updated contact details, social profiles, and business insights.
- API Integration: Use APIs to connect Salesforce with external systems to pull in relevant and up-to-date data automatically.
6. Standardization and Normalization
- Field Standardization: Standardize data fields to ensure uniformity in data entry, such as using consistent date formats, country codes, and phone number formats.
- Normalization Processes: Normalize data to eliminate redundancies and inconsistencies, ensuring that data is presented in a consistent and usable format.
7. Data Monitoring and Reporting
- Dashboards and Reports: Create dashboards and reports to monitor data quality metrics such as completeness, accuracy, and consistency.
- Alerts and Notifications: Set up alerts to notify data stewards or administrators of data quality issues that require immediate attention.
8. Regular Data Maintenance
- Scheduled Cleanups: Implement scheduled data cleanup activities, including deduplication, validation, and enrichment tasks, to maintain data quality over time.
- Archiving: Archive old or inactive data that is no longer relevant to reduce clutter and improve system performance.
9. User Training and Engagement
- Training Programs: Conduct regular training sessions for Salesforce users to educate them on the importance of data quality and proper data entry techniques.
- User Feedback: Encourage users to report data issues and provide feedback on data quality, fostering a collaborative approach to data management.
10. Advanced Data Quality Solutions
- AI and Machine Learning: Utilize AI and machine learning tools to identify patterns, predict data quality issues, and automate Salesforce Data Cleansing tasks.
- Data Quality Platforms: Invest in comprehensive data quality management platforms that offer a suite of tools for cleansing, enrichment, and governance.
11. Data Migration and Integration Management
- Migration Best Practices: When migrating data into Salesforce from other systems, follow best practices to ensure data integrity, including thorough data mapping and validation.
- Integration Validation: Regularly validate data integrations to ensure that data flowing between Salesforce and other systems remains accurate and consistent.
12. Ongoing Improvement and Adaptation
- Continuous Improvement: Adopt a culture of continuous improvement where data quality practices are regularly reviewed and updated to adapt to changing business needs and technological advancements.
- Benchmarking: Benchmark data quality metrics against industry standards to identify areas for improvement and measure progress.
Best Practices for Improving Data Accuracy
Improving Salesforce Data Cleansing accuracy is critical for organizations to make informed decisions, enhance operational efficiency, and maintain competitive advantage. Here are comprehensive best practices for improving data accuracy:
1. Data Governance
- Define Clear Policies: Develop comprehensive data governance policies that define standards for data quality, data entry, and data usage.
- Assign Responsibilities: Designate data stewards or data owners who are accountable for maintaining data accuracy.
- Compliance and Auditing: Implement regular compliance checks and audits to ensure adherence to data governance policies.
2. Standardize Data Entry
- Data Entry Standards: Standardize data formats for all key fields such as dates, addresses, and phone numbers to ensure uniformity.
- Mandatory Fields: Use mandatory fields to ensure essential data is captured at the point of entry.
- Validation Rules: Implement validation rules in data entry forms to enforce data standards and prevent incorrect entries.
3. Use Automated Tools
- Data Validation Tools: Utilize automated tools that validate data in real-time as it is entered into the system.
- Deduplication Tools: Implement deduplication software to identify and merge duplicate records.
- Error Detection: Use automated error detection tools to identify and correct data inaccuracies.
4. Salesforce Data Cleansing Processes
- Regular Data Audits: Conduct regular data audits to identify inaccuracies, inconsistencies, and outdated information.
- Scheduled Cleansing: Establish scheduled Salesforce Data Cleansing routines to continuously correct and update data.
- Corrective Actions: Develop and follow a clear process for addressing identified data issues.
5. Data Enrichment
- Third-Party Data: Integrate third-party data sources to enrich and validate existing data.
- API Integrations: Use API integrations to automatically update and enrich data with accurate and up-to-date information.
- Verification Services: Employ data verification services to confirm the accuracy of key data fields such as contact information and addresses.
6. Training and Awareness
- User Training: Provide regular training sessions for employees on the importance of data accuracy and best practices for data entry.
- Awareness Programs: Create awareness programs to emphasize the impact of accurate data on business outcomes.
- User Guidelines: Develop and distribute clear data entry guidelines to all users.
7. Implement Data Quality Metrics
- Define Metrics: Define key data quality metrics such as accuracy, completeness, consistency, and timeliness.
- Monitor Performance: Use dashboards and reports to monitor data quality metrics regularly.
- Continuous Improvement: Implement a continuous improvement process based on data quality metrics to identify and address areas for improvement.
8. Optimize Data Integration
- Consistent Data Models: Ensure consistent data models across integrated systems to maintain data integrity.
- Data Mapping: Perform detailed data mapping when integrating systems to ensure data accuracy is maintained.
- Synchronization: Implement robust synchronization processes to keep data consistent across multiple systems.
9. Archiving and Deletion Policies
- Archiving Strategies: Develop archiving strategies to store historical data while keeping the current data set clean and relevant.
- Deletion Policies: Implement policies for the timely deletion of obsolete or irrelevant data to maintain data accuracy.
- Data Lifecycle Management: Manage the entire data lifecycle, from creation to deletion, ensuring data accuracy at each stage.
10. Engage in Continuous Feedback
- User Feedback: Encourage users to report data inaccuracies and provide feedback on data quality.
- Stakeholder Involvement: Involve key stakeholders in data quality initiatives to ensure that the data meets their needs and expectations.
- Iterative Improvements: Use feedback to drive iterative improvements in data accuracy practices.
11. Advanced Technologies
- Machine Learning: Use machine learning algorithms to identify patterns and predict potential data inaccuracies.
- Artificial Intelligence: Implement AI-driven Salesforce Data Cleansing and enrichment tools to automate data accuracy improvements.
- Data Quality Platforms: Invest in comprehensive data quality management platforms that provide a suite of tools for ensuring data accuracy.
12. Review and Adapt
- Regular Reviews: Conduct regular reviews of data accuracy practices to ensure they remain effective and relevant.
- Adapt to Changes: Be agile and adapt data accuracy strategies to changes in business processes, regulatory requirements, and technological advancements.
- Benchmarking: Benchmark data accuracy against industry standards and best practices to identify areas for improvement.
Conclusion: Salesforce Data Cleansing
Salesforce Data Cleansing is essential for maintaining accurate, reliable, and efficient CRM operations. It involves identifying and correcting inaccuracies, updating outdated information and removing duplicates. Salesforce Data Cleansing improves decision-making, enhances customer interactions, and optimizes marketing and sales strategies.
Key strategies include establishing robust data governance policies, using automated tools for validation and deduplication, and implementing regular data audits and enrichment processes. Standardizing data entry, leveraging third-party data sources, and training users on best practices are also critical.
Data governance ensures that there are clear roles and responsibilities, while automated tools and regular audits help maintain data quality over time. Training users and standardizing data entry prevent common errors, ensuring consistent and accurate data input. Integrating third-party data and employing advanced technologies like AI and machine learning can further enhance data accuracy and efficiency.